Using AI to Unlock the Value of your Data - Managing Non-Performing Loans (NPLs)
6 Minute Read - Last month I got the opportunity to give a Special Address presentation at Finovate Europe; a conference showcasing cutting-edge banking and financial technology through a unique blend of short-form demos and key insight presentations from thought-leaders. The following is overview of my talk. All thoughts and views are my own.
Challenges Becoming Data-Driven
Studies have shown that the majority of companies who understand their data, obtain true benefits and value as a result of being able to make more informed decisions along with the ability to adapt and respond to changes quickly. In one of my previous articles “The Analytics Summit 2021 Trends - Data, Sustainability & Ethics”, I mentioned that only 37% of the 85% of businesses that aim to be data-driven are successful. The reason why so many of these businesses fail, is due to a number of challenges when it comes to data collection and usage:
- Objective Identification: It can be difficult to combine the right people and knowledge in order to understand the value of the data in alignment with the strategic direction of the business.
- Secure Accessibility & Integration: During business growth, data systems can sometimes become disjointed due to the expansion in the various applications, services, and databases, making it hard to integrate and centralise the data.
- Cultural Resistance: Humans are a creature of habit, so introducing new procedures and processes can often result in some sort of adoption hesitancy.
- Data Overload & Trust: Consolidating and validating data being retrieved from a range of difference sources can be difficult, and often results in the valuable data not being utilised.
However, the companies who are successful in overcoming these challenges, show a number of common traits such as:
- Persistence: They have firm continuation in their course despite any difficulties or obstacles.
- Resilience: They have an ability to adapt and recover from setbacks or failures.
- Great Execution: They put a plan in place, provide the necessary resources, and then drive towards execution.
Leveraging Artificial Intelligence (AI)
Considering these challenges outlined above, many companies look to leverage a technical solution to get a solid grasp of the value within their data. AI is a prime candidate to supercharge human thinking when it comes to understanding and unlocking data value by enabling patterns to be recognised, contexts to be visualised, and more informed decisions to be made. A recent study showed that 75% of executives fear going out of business within five years if they don’t utilise AI to understand their data (Semrush, 2021). But there are also a number of industry challenges associated with businesses trying to adopt AI. These come down to understanding the business value, identifying appropriate use-cases, applying reasonable investment to realise potential, employing the correct people with the right skills and expertise, and also the challenges associated with collecting and structuring the data, all of which I discussed in more detail in a recent article “Industry Future of AI for Health and Wellness”.
Financial Services
One industry that relies heavily on data to drive informed decision-making is financial services. For example, if you look at asset management, contract intelligence, and due diligence, they all have their own individual problems and tackle them in their own individual way, but one challenge that’s common across them all is that they need to ingest large amounts of documents and large volumes of data in a short space of time. Some of these documents may have been digitised 10-15 years ago when many laws and structures were different, in some cases these documents may not have been scanned to the highest quality, and a high majority of these documents contain unstructured data where it can be really difficult to use automated approaches to define and extract the key and relevant pieces of information. A recent study estimated that 85% of all data residing in an organisation is unstructured (expected to grow 2x in next couple of years) and only 2% of this data is being utilised in a meaningful way (Datamation, 2017). So understanding the data within these documents is a real challenge.
Non-Performing Loans (NPL)
One focus within the financial services industry that can really benefit from understanding their data is NPLs. An NPL, which traditionally follows the trajectory pattern outlined below, is where the borrower has failed to make any of the scheduled payments, normally within 90 days. Looking at the current crisis that started at the beginning of 2020 due to the Coronavirus, should the NPLs follow the same pattern (i.e. the mean time from the start of the crisis to the peak of the NPL ration being 3.3 years), the NPL peak could be reached in the first half of 2023.
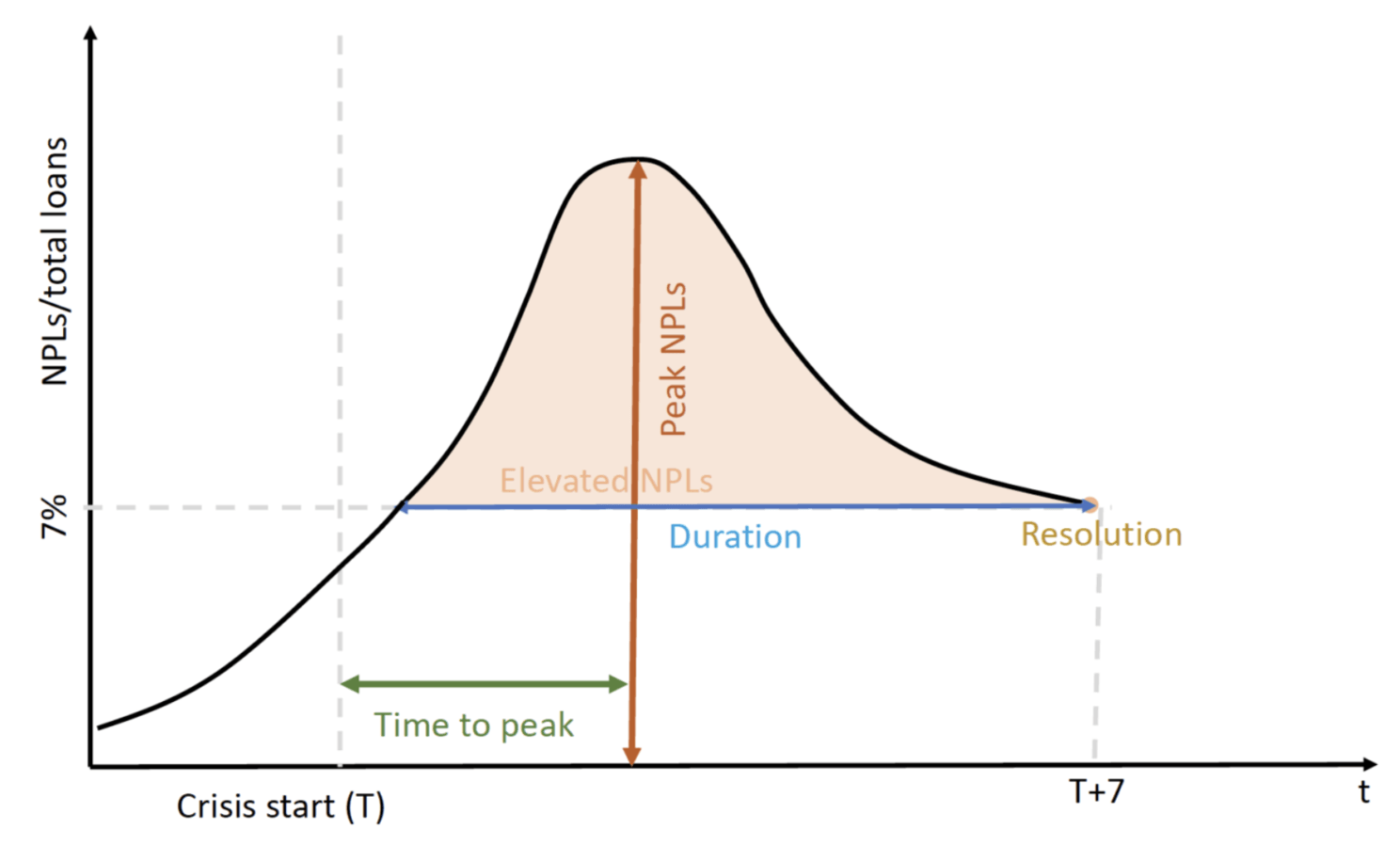
When this happens, banks normally group these NPL portfolios into batches and put these out for tender – with the aim to sell them to the likes of secondary banks and asset managers in order to free up capital and/or focus on performing loans that bring in income. (Investopedia, 2021). During this bidding process, the data contained within all these NPLs (potentially millions of pages) will need to be analysed and value identified in order for the bidders to make an appropriate bidNew Paragraph.
Current Approach (Manual Processing)
At the moment, this portfolio exploration is a very manual process that is time consuming, expensive and prone to errors. A typical loan portfolio may contain hundreds of unstructured documents which are inconsistent and can vary widely based on originator, vintage, or even scanned image quality. Resources are required to manually read through these millions of data points, and although we sometimes like to think that we are perfect, humans make mistakes. After a certain amount of time reading through these documents with varying levels of quality, tiredness will start to kick in and the monotonous nature of performing the same task over and over will start to introduce errors and inaccuracies such as 3’s becoming 8’s, 5’s becoming 6’s, and a decimal point being shifted. So when the NPL peak hits (estimated to be Q1/Q2 of 2023), companies can either choose to spend a lot of time, money, and resources, or they could just use the solution that we have developed.
Altada’s Document Intelligence Platform
In order to ensure that decisions are not being made using this inconsistent data, we have developed an AI document intelligence platform that is trained to catalogue, extract, and understand key data within loan portfolios. Our unique solution utilises a combination of technologies such as object character recognition (OCR) to extract the key components of the document, natural language processing (NLP) to understand the context on that content, and a range of machine learning (ML) and AI techniques to categorise and analyse the data. For an overview of this platform, please check out our Document Intelligence AI demo video.
In a recent statement, Jason Pinson who is CEO of Rocktop Partners, a large investment manager outlined that “Altada’s AI solutions have given our business the competitive edge in the market with transformational operational and cost efficiencies, such as the reduced cost of processing a loan file from $400 to $25, and reducing the time it takes to process a portfolio from six to eight weeks to 48 hours” (Silicon Republic, 2021). But not only has our platform shown to reduce costs by 90%, increase efficiencies by 20x, and achieve over 90% accuracy, it primarily allows companies to make quicker and more informed decisions enabling their money to start working for them in a shorter timeframe.
If you want to know more and understand how out platform can revolutionise your process, make sure to get in touch. Until next time, I hope you enjoyed the read. GB
Hashtags
#AI #ArtificialIntelligence #DataScience #MachineLearning #ML #Ethics #Data #DocumentIntelligence #OCR #NLP #NPL #Finance
References
- Datamation. (2017). “Unstructured Data”. Available at: https://www.datamation.com/big-data/unstructured-data. [Accessed 21 April 2022]
- Forbes. (2022). “Leading Founders Of Fast-Growing Companies Share Three Tenets Of Success”. Available at: https://www.forbes.com/sites/benjaminlaker/2022/04/19/founders-of-fast-growing-companies-share-three-tenets-of-success/. [Accessed 21 April 2022]
- International Banker. (2021). “TACKLING NON-PERFORMING LOANS IN A PERFORMING ECONOMY”. Available at: https://internationalbanker.com/banking/tackling-non-performing-loans-in-a-performing-economy/. [Accessed 21 April 2022]
- Investopedia. (2021). "Nonperforming Loan (NPL)". Available at: https://www.investopedia.com/terms/n/nonperformingloan.asp. [Accessed 21 April 2022]
- Semrush. (2021). “65 Artificial Intelligence Statistics for 2021 and Beyond”. Available at: https://www.semrush.com/blog/artificial-intelligence-stats/. [Accessed 21 April 2022]
- Silicon Republic. (2021). “Cork start-up Altada to expand globally following $11.5m investment”. Available at: https://www.siliconrepublic.com/start-ups/altada-cork-start-up-jobs-ai-series-a-funding. [Accessed 21 April 2022]
- Think Evolve Solve. (2021). “CHALLENGES ORGANISATIONS FACE IN BECOMING DATA-DRIVEN". Available at: https://thinkevolvesolve.ie/challenges-organisations-face-in-becoming-data-driven/. [Accessed 8 March 2022]
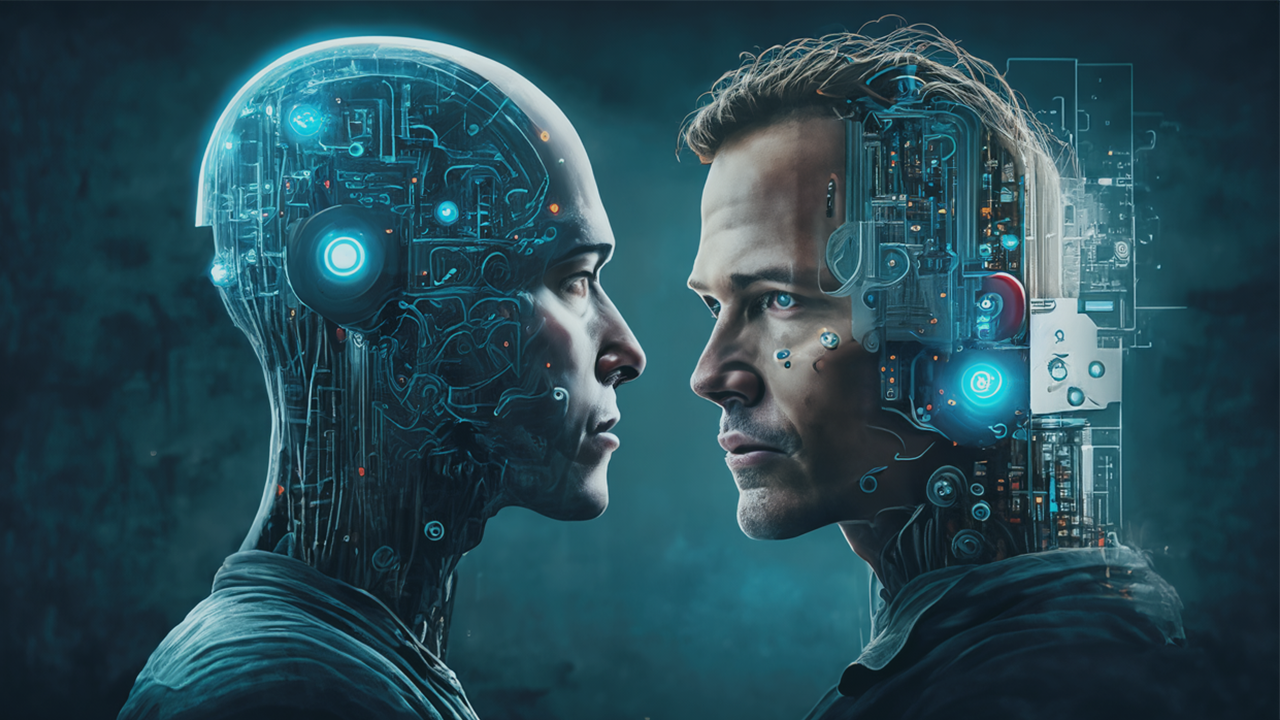
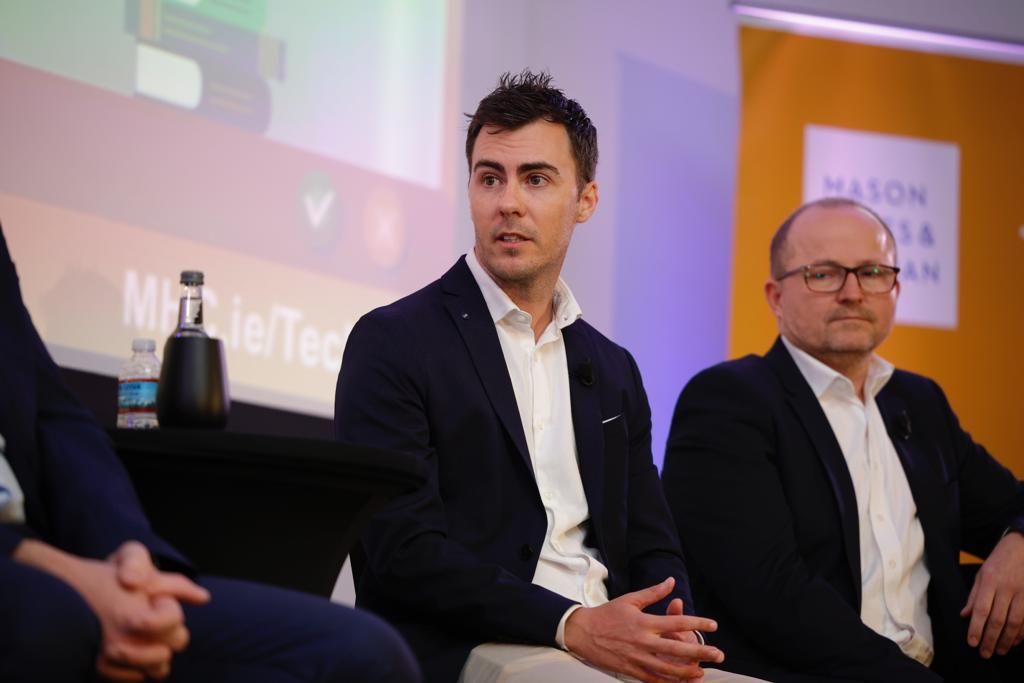
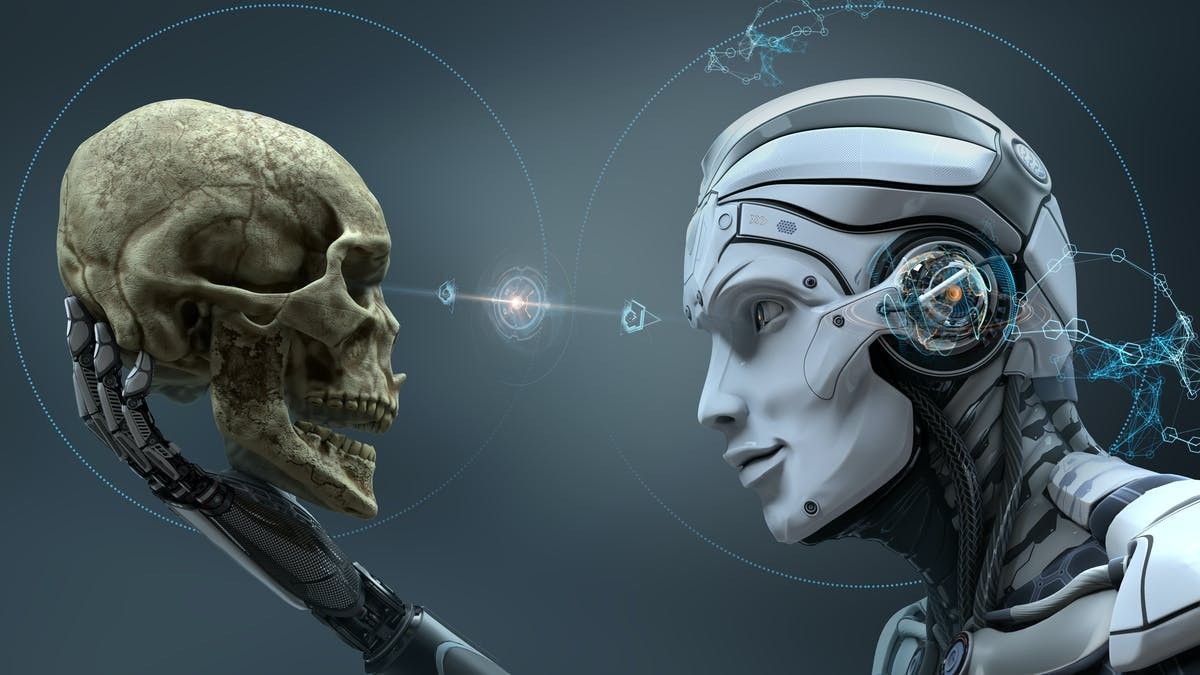
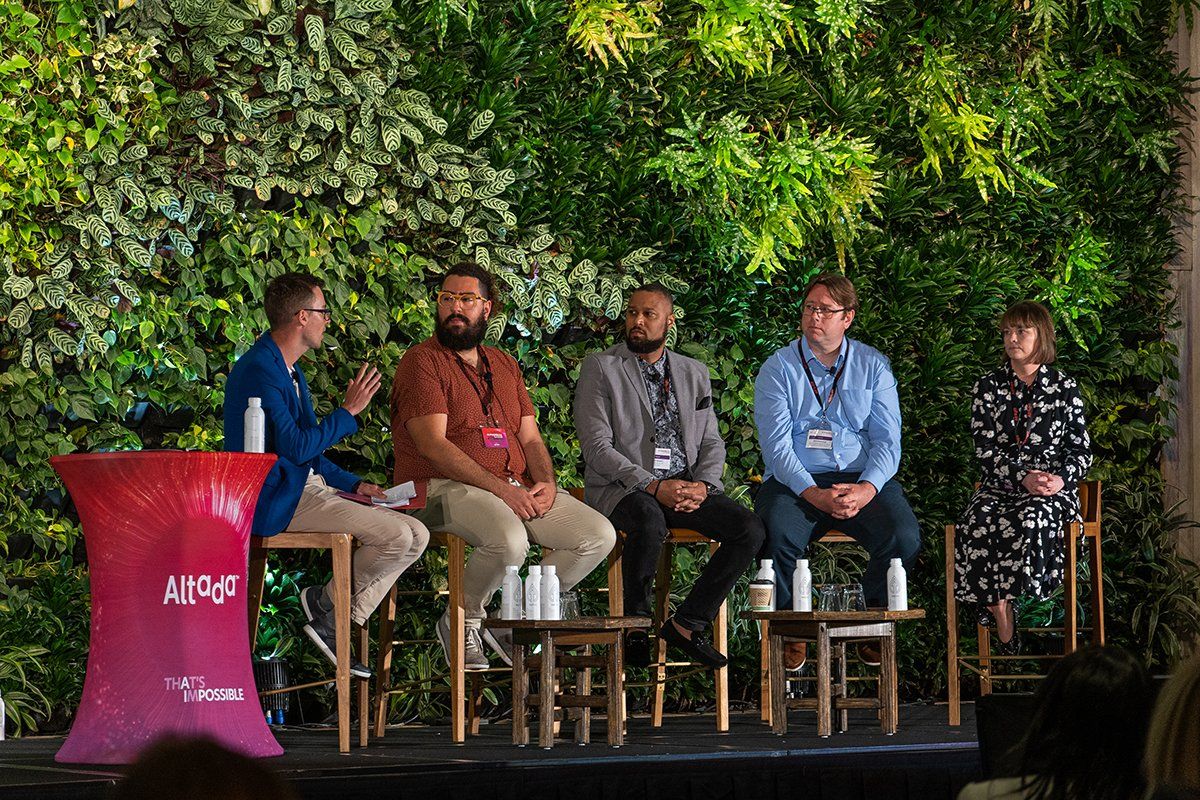
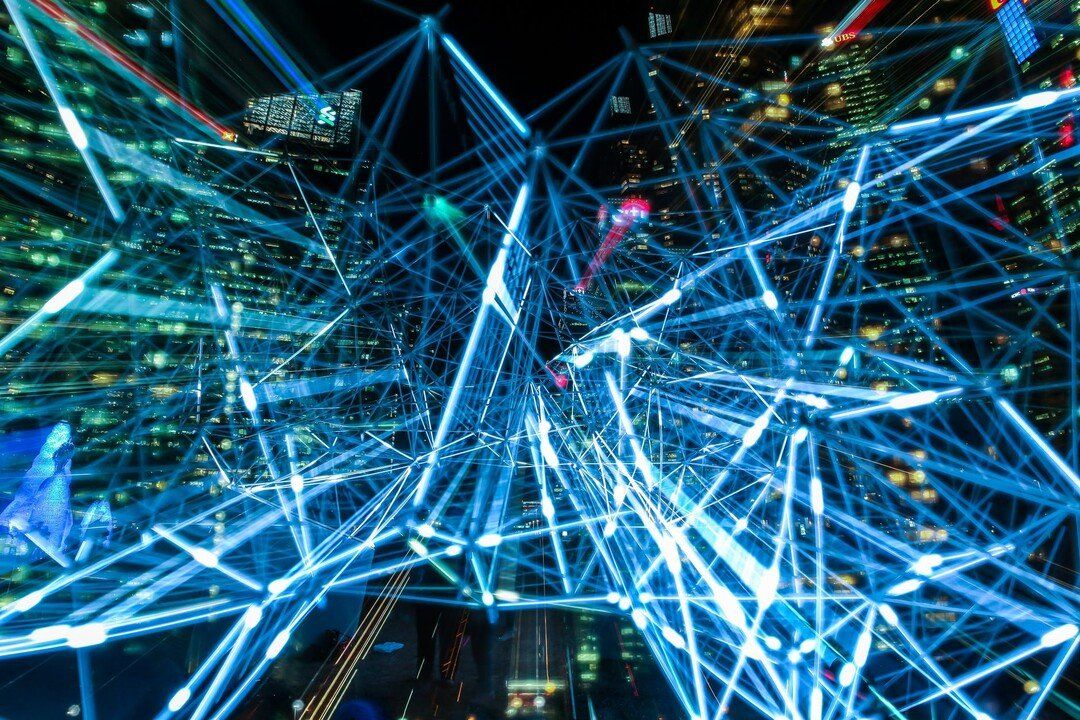
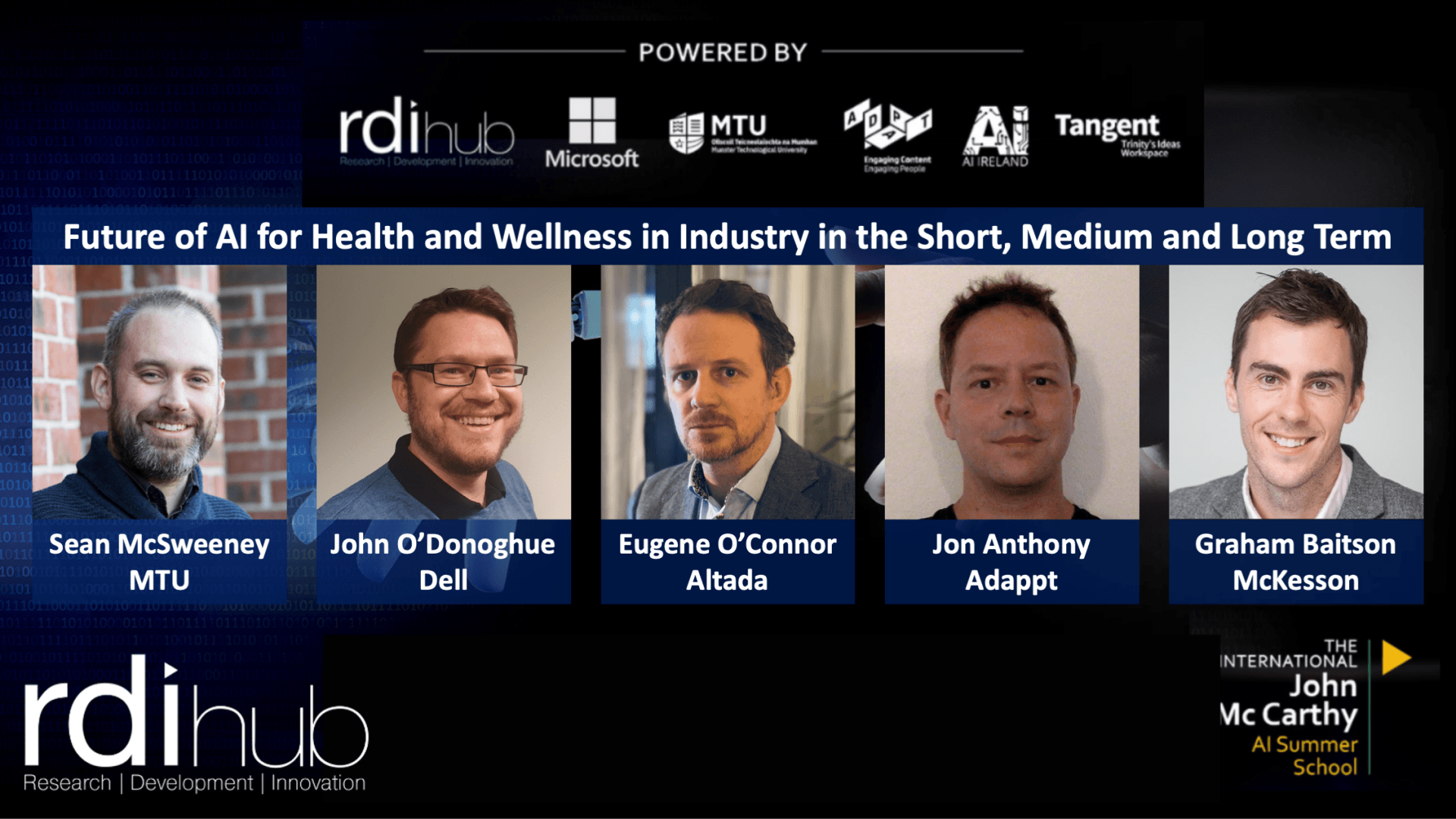
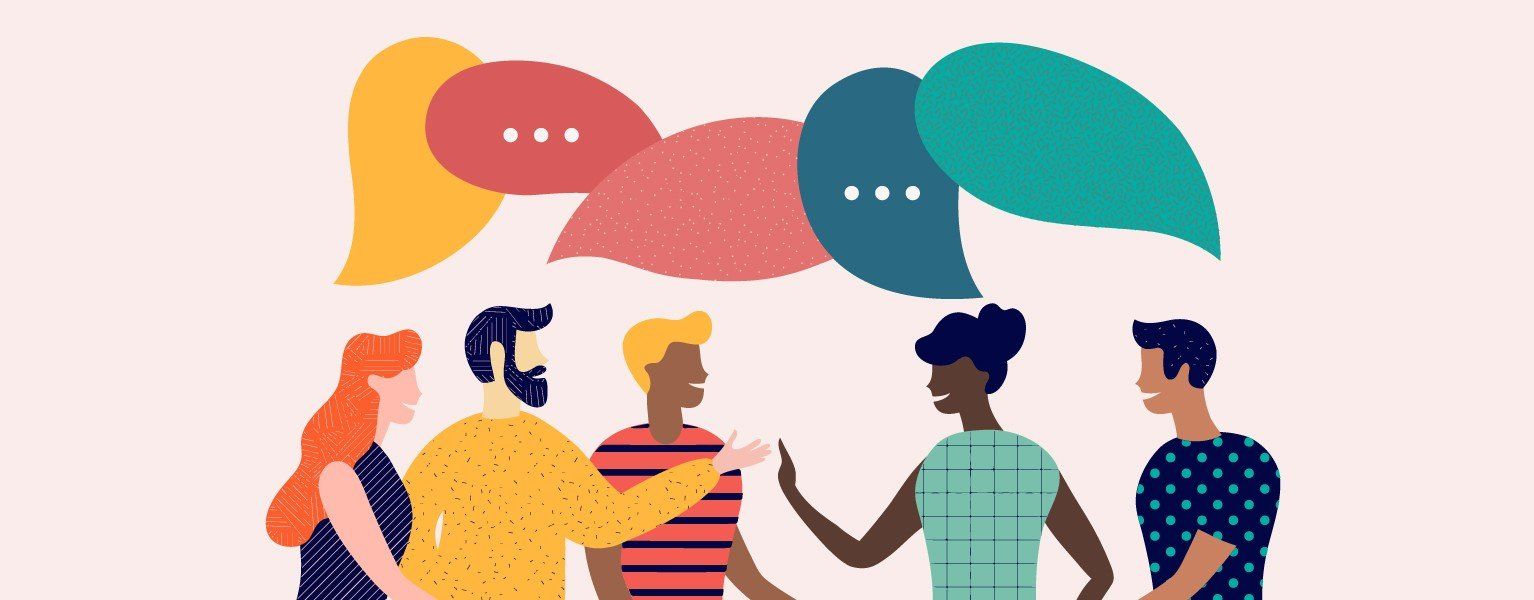
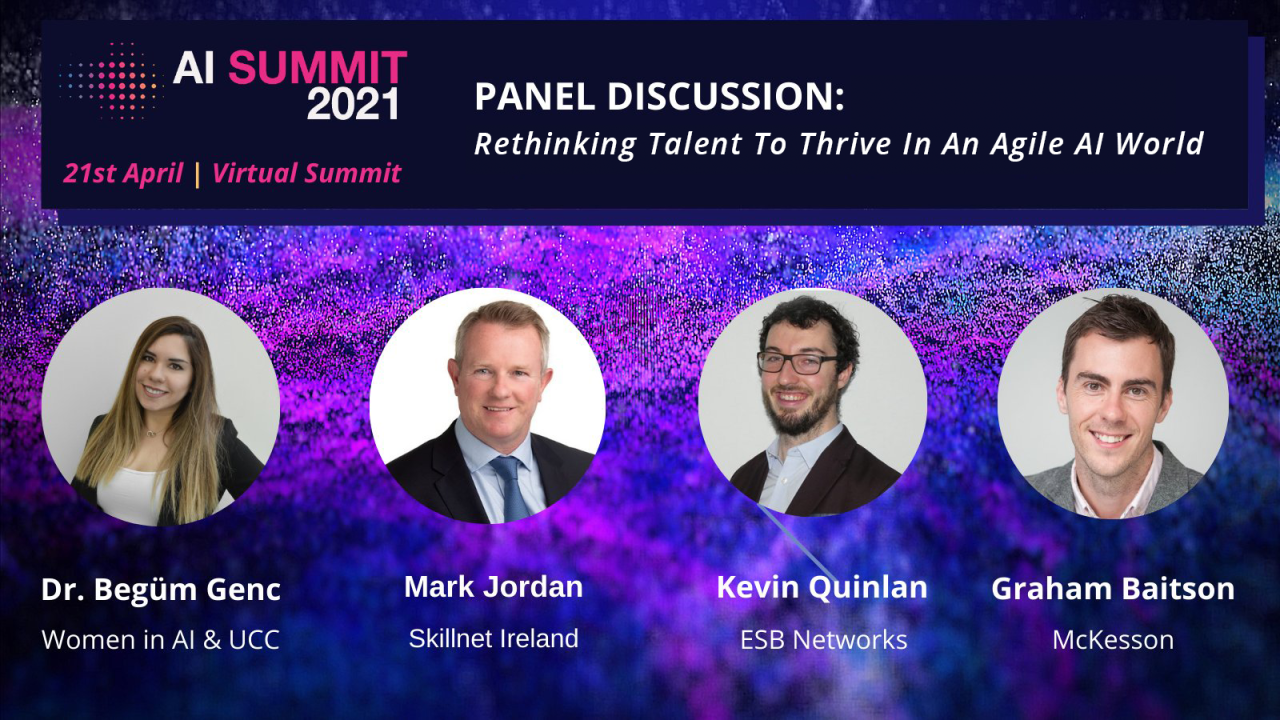
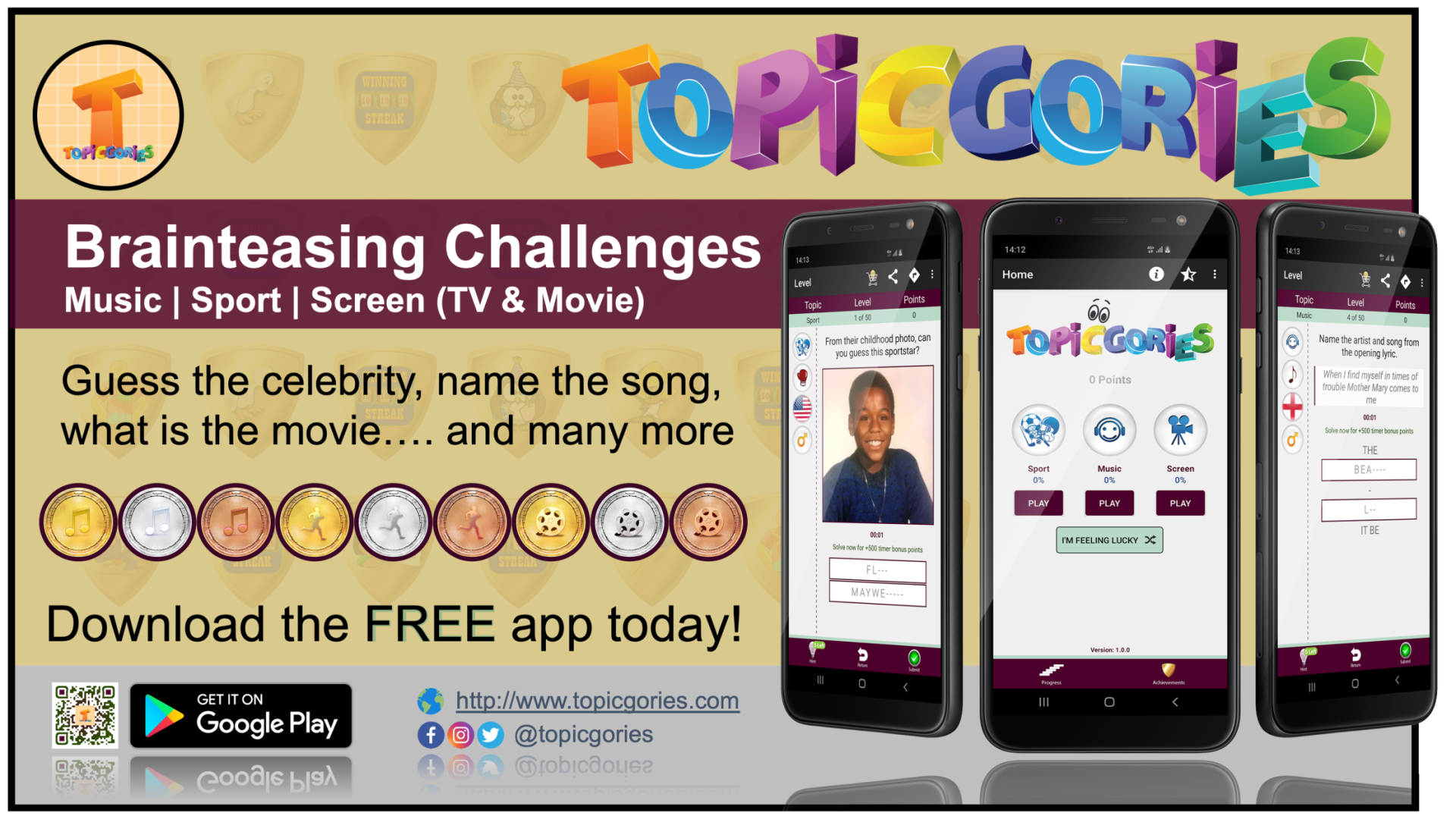
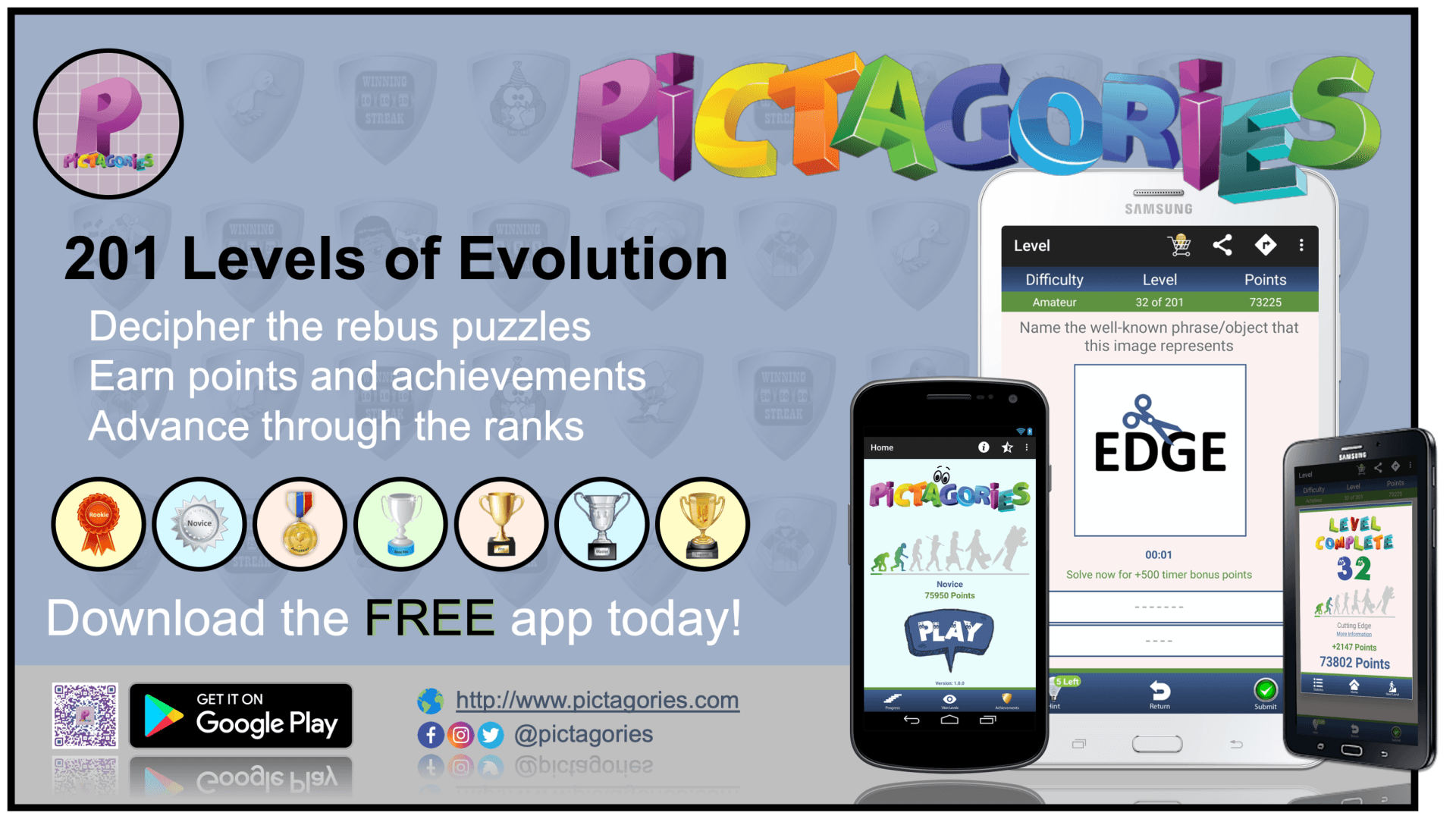