Microsoft Paint – For the AI Generation
3 minute read
One of the many talents that I wish I had and unfortunately was not blessed with is the ability to draw realistically. As a kid, I was obsessed with colouring books, patterns and drawing. I used to record the TV screen using the VHS video player (yes this was before the dawn of Netflix and DVD) and then try to recreate what was on the screen. Needless to say, none of them were any good and I don’t have any masterpieces hanging in any galleries around the world. But, with the help of the latest developments from Nvidia Research, I now get to see my ’masterpieces’ come to life.
GauGAN – From Doodles to Works of Art
This week at the Nvidia GPU Technology Conference (GTC) 2019, Nvidia unveiled their latest image processing research efforts, and it’s pretty incredible. Trained using 1 million images on Flickr (Burns, M., 2019) and leveraging generative adversarial networks (GANs), the deep learning model transforms basic segmentation maps into highly realistic images.
Video 01: GauGAN
The software allows you to draw an images using basic shapes, and then with the inclusion of labelling, be able to transform those shapes into realistic landscape imagery. In addition, it also has the built-in awareness of what has been generated within the scene, allowing the image to dynamically adapt to the other textures. For example, if you have an image of a green leafy tree on a summers day and then label the ground as snow, the tree will convert to barren. If you then introduce a lake, the tree and nearby elements will automatically be reflected in the water.
“ It’s like a coloring book picture that describes where a tree is, where the sun is, where the sky is…and then the neural network is able to fill in all of the detail and texture, and the reflections, shadows and colors, based on what it has learned about real images” - Bryan Catanzaro, VP of Applied Deep Learning Research, Nvidia
Going, Going, GAN
Invented in 2014, GANs contain the potential to learn the natural features of a dataset, resulting in the ability to imitate any distribution of data. The concept takes two cooperating neural networks; a generator and a discriminator. The Discriminator is initially trained on real data to produce a blueprint of what a real image should look like. The Generator then produces new data instances and presents them to the discriminator. The Discriminator then evaluates the authenticity by comparing the sample to its blueprint. The Discriminator provides feedback to the Generator on how to improve the realism of its synthetic images. Both work together to improve each other’s function (i.e. Generator is improving at creating realistic images and Discriminator is improving at detecting them).
Virtual Worlds
This approach has the potential to completely transform the creation of scenic environments. Not only does allow people who may not be highly skilled start creating realistic scenery, but also allows the professionals to focus more of their efforts on prototyping more interesting sceneries and making quicker changes more efficiently. This powerful tool could have potential applications in architecture, landscaping, game designing and even film making. Although GauGAN at present focuses purely on natural elements like rocks, land and sea, “the underlying neural network is capable of filling in other landscape features, including buildings, roads and people” (Nvidia, 2019). With over 170 full-time researchers and in 2018, rolling out 104 publications, 51 patent applications and 12 open-source software packages (Medium, 2019), I really interested to see what further developments are made in this space.
More Information
The following are more links if you need further information
- NVidias’ AI Playground: https://www.nvidia.com/en-us/research/ai-playground/
- GauGAN Project Website: https://nvlabs.github.io/SPADE/
- GauGAN Research Paper: https://arxiv.org/abs/1903.07291
- GauGAN Code: https://github.com/NVLabs/SPADE
References
- Burns, M., (2019). Fdsfds. Available at: https://techcrunch.com/2019/03/18/nvidia-ai-turns-sketches-into-photorealistic-landscapes-in-seconds/. [Accessed 20 March 2019].
- Figure Cover: https://blogs.nvidia.com/blog/2019/03/18/gaugan-photorealistic-landscapes-nvidia-research/
- Medium (2019). GTC 2019 | NVIDIA’s New GauGAN Transforms Sketches Into Realistic Images. Available at: https://medium.com/syncedreview/gtc-2019-nvidias-new-gaugan-transforms-sketches-into-realistic-images-a0a74d668ef8. [Accessed 20 March 2019]
- Nvidia (2019). Stroke of Genius: GauGAN Turns Doodles into Stunning, Photorealistic Landscapes. Available at https://blogs.nvidia.com/blog/2019/03/18/gaugan-photorealistic-landscapes-nvidia-research. [Accessed 19 March 2019].
- Video 01: GauGAN. Available at: https://youtu.be/p5U4NgVGAwg. [Accessed 19 March].
- Wolfe, J. (2019). Stroke of Genius: NVIDIA Researchers Debut GauGAN at GTC 2019. Available at: https://www.awn.com/news/stroke-genius-nvidia-researchers-debut-gaugan-gtc-2019. [Accessed 20 March 2019].
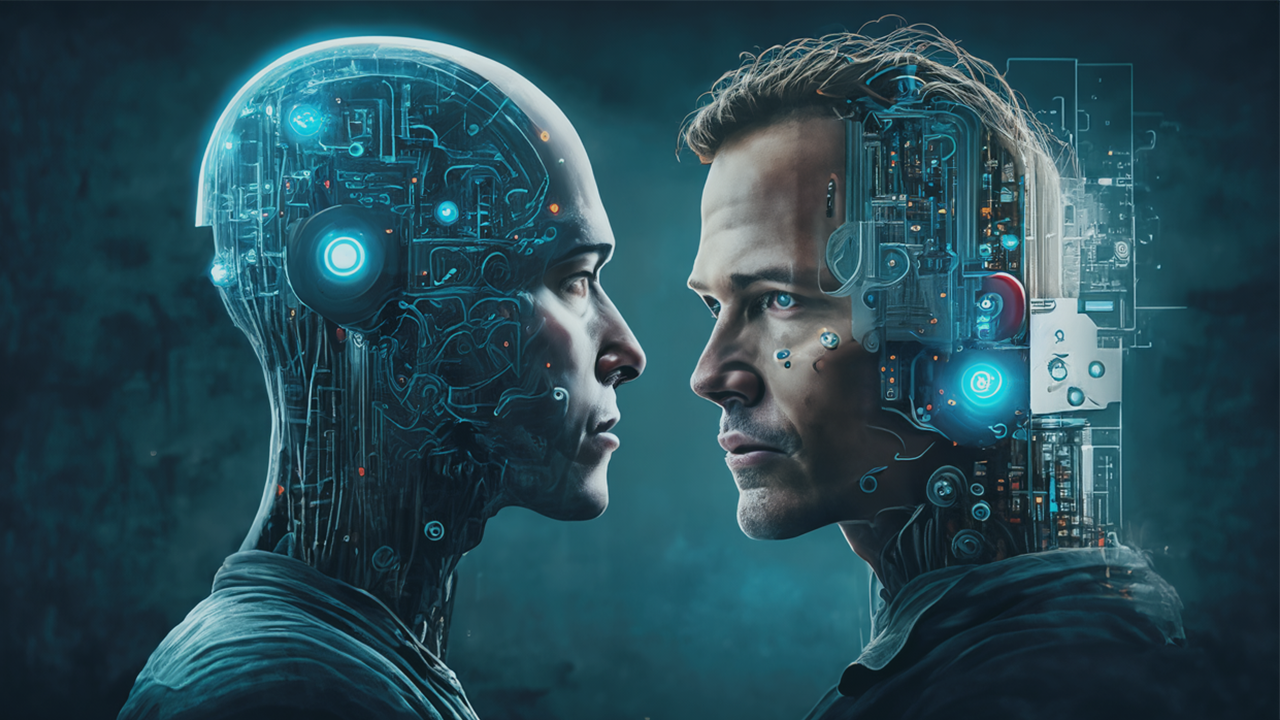
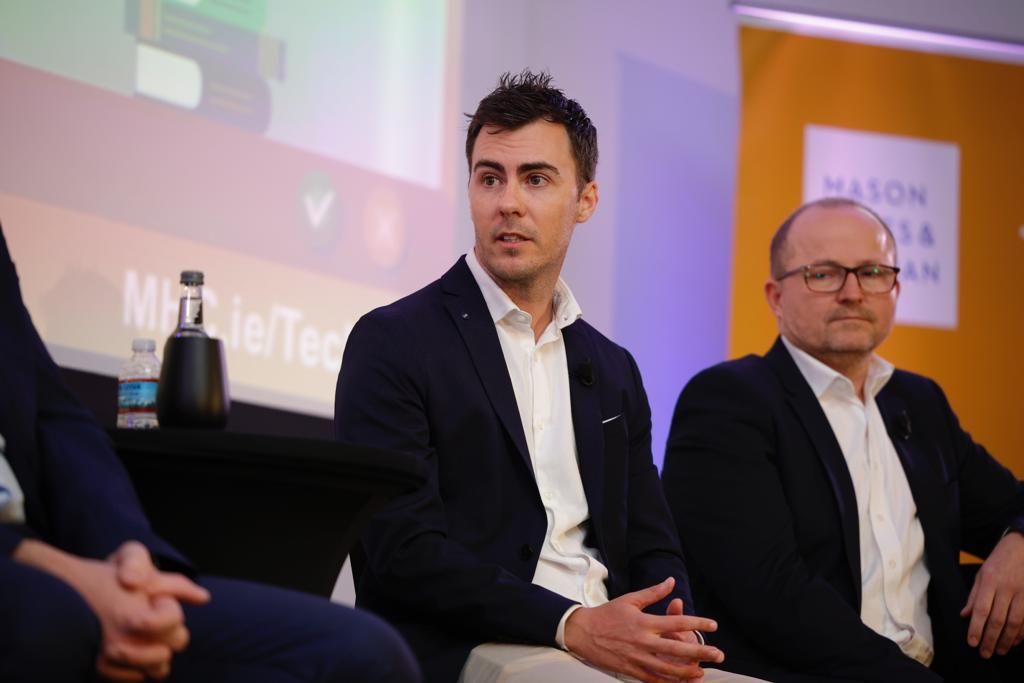
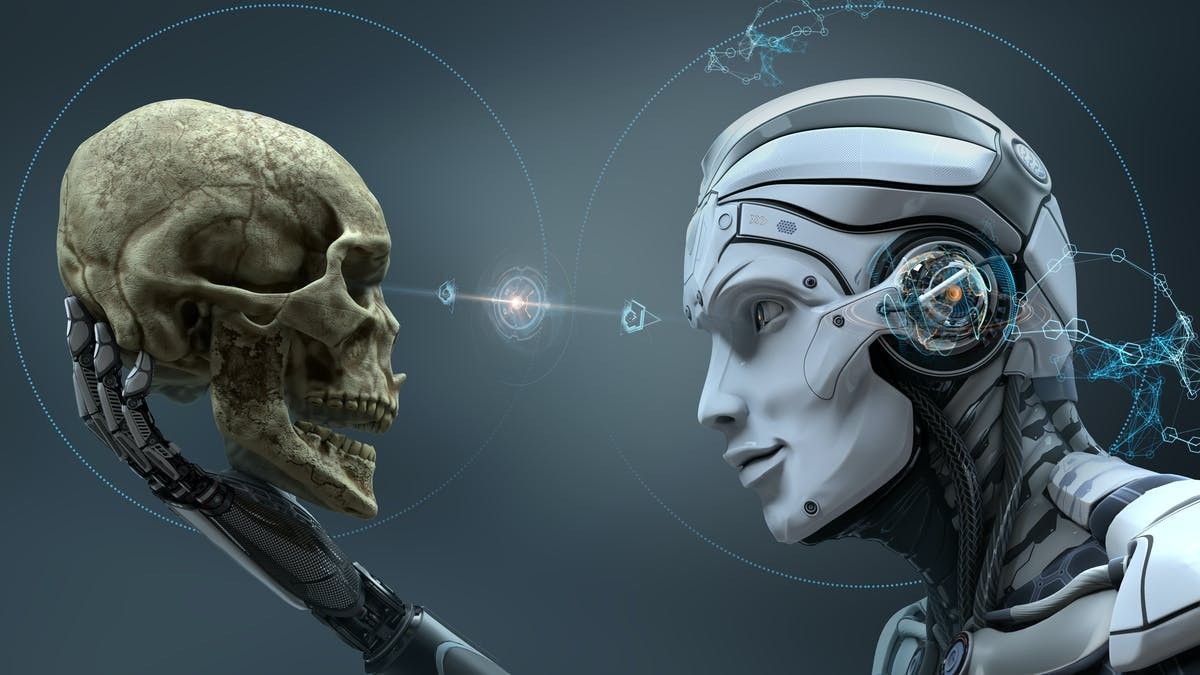
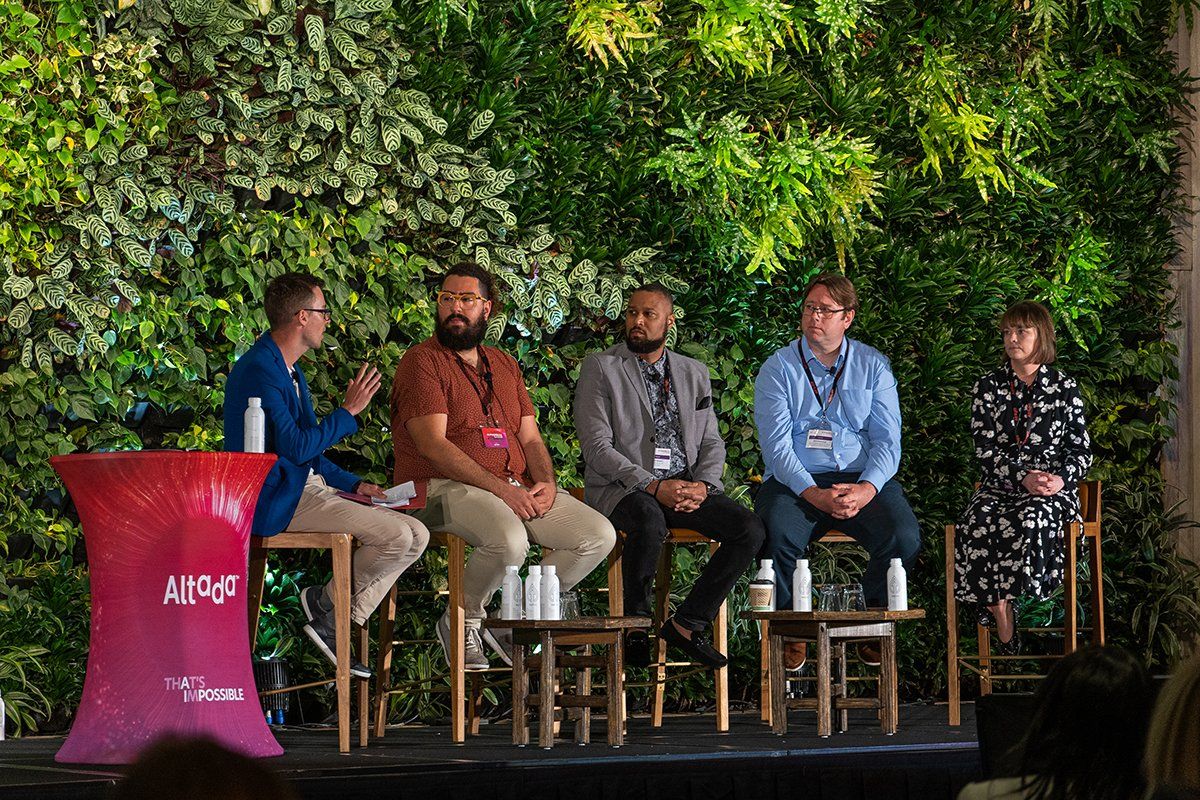
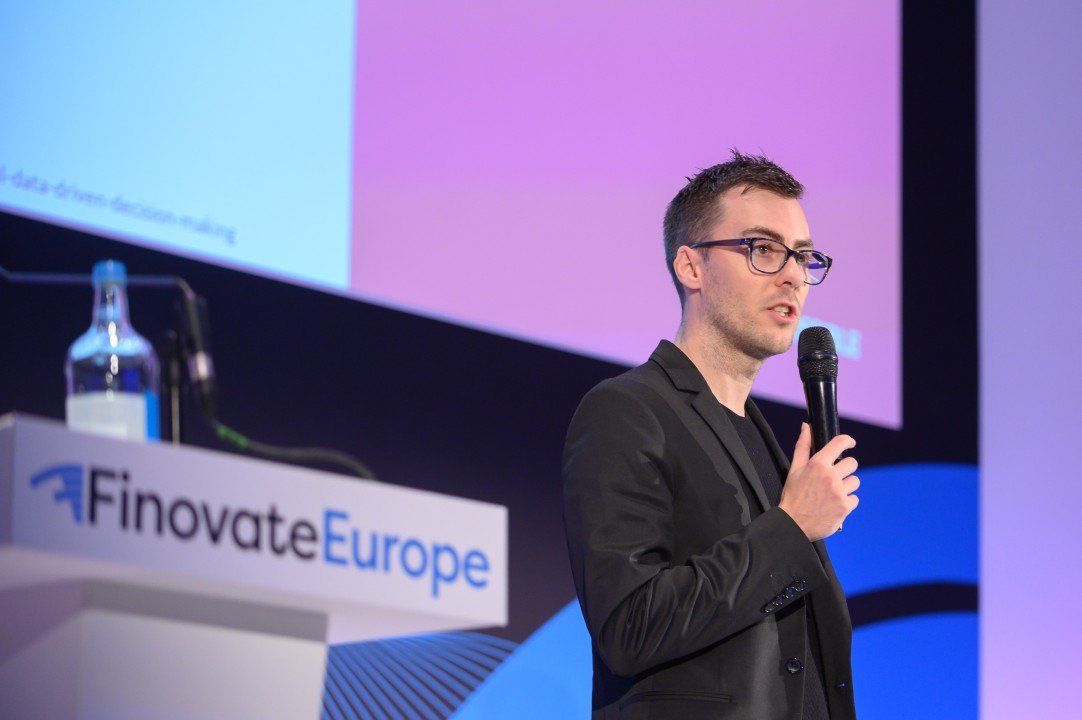
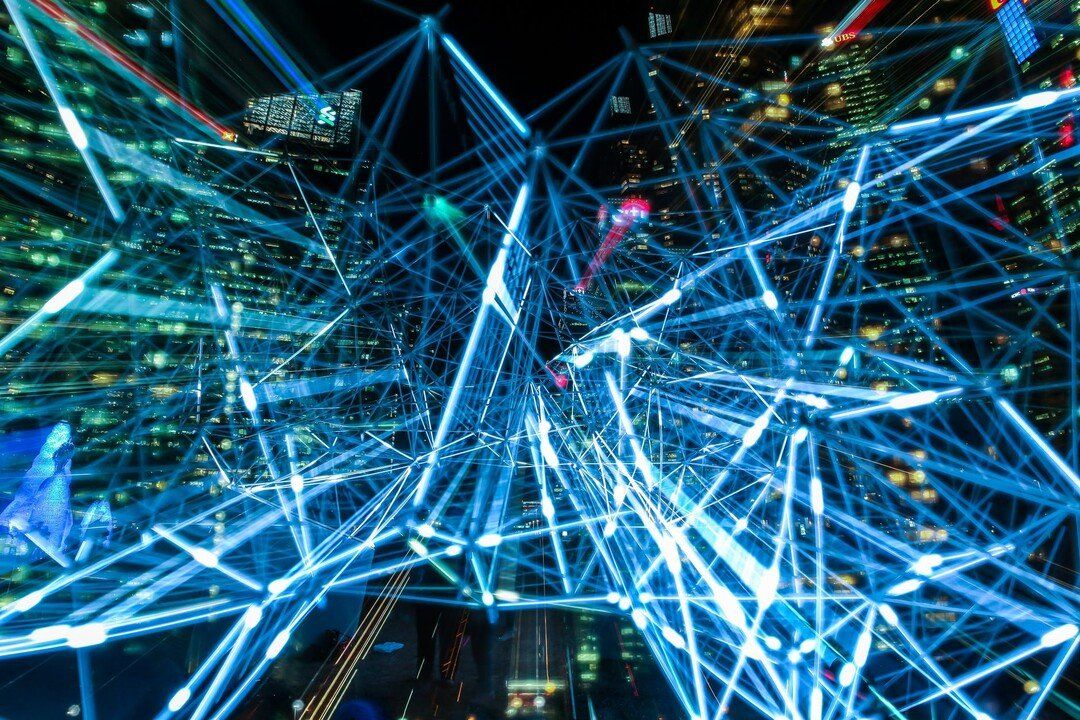
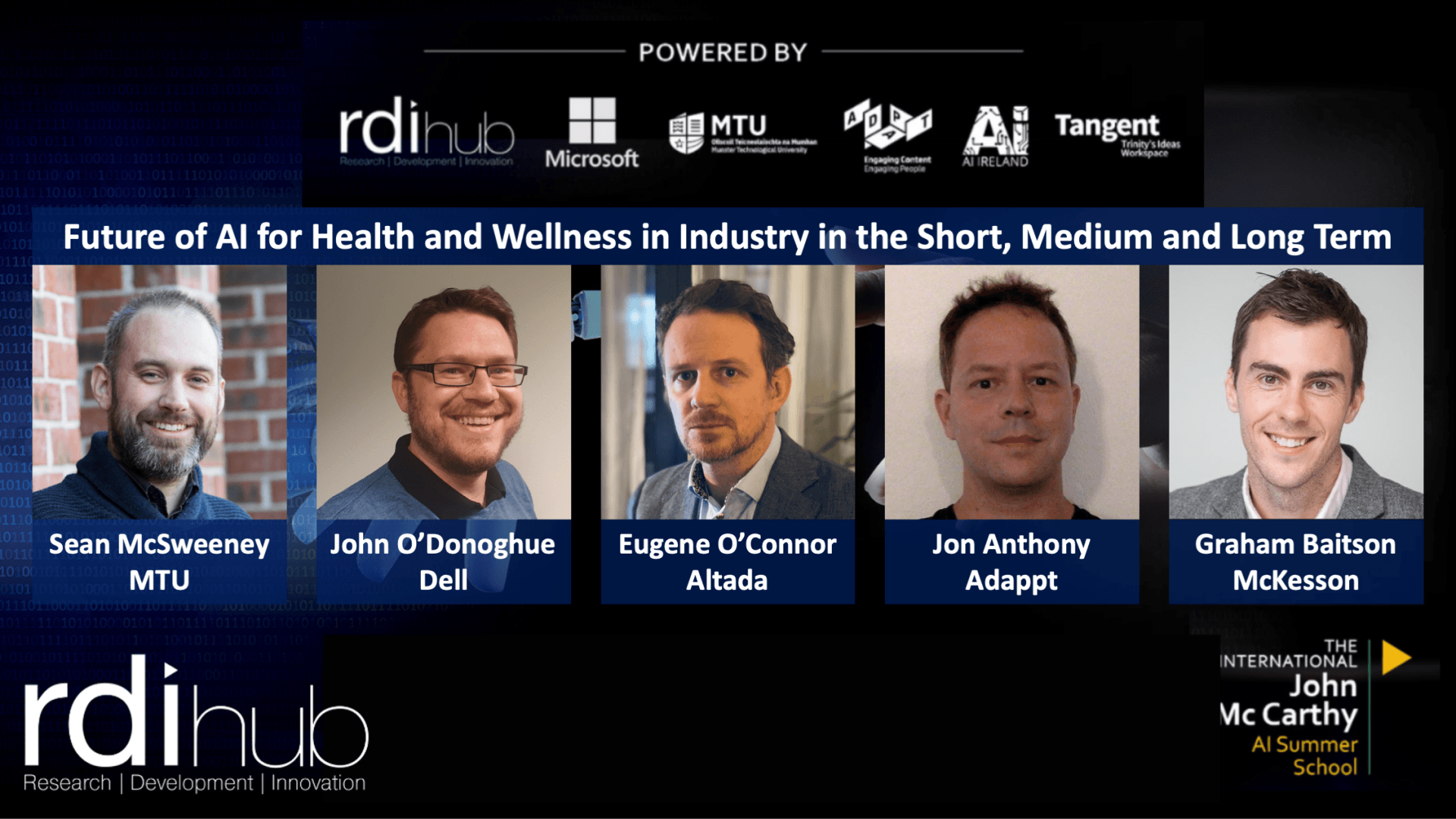
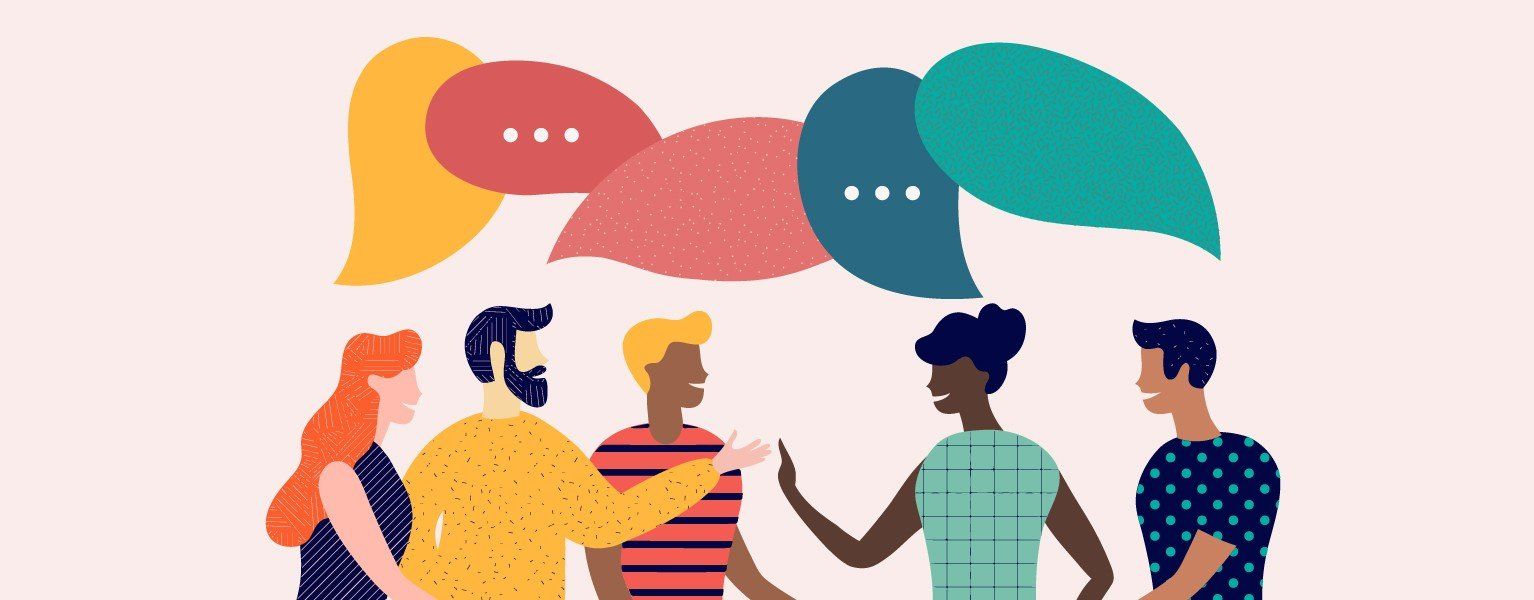
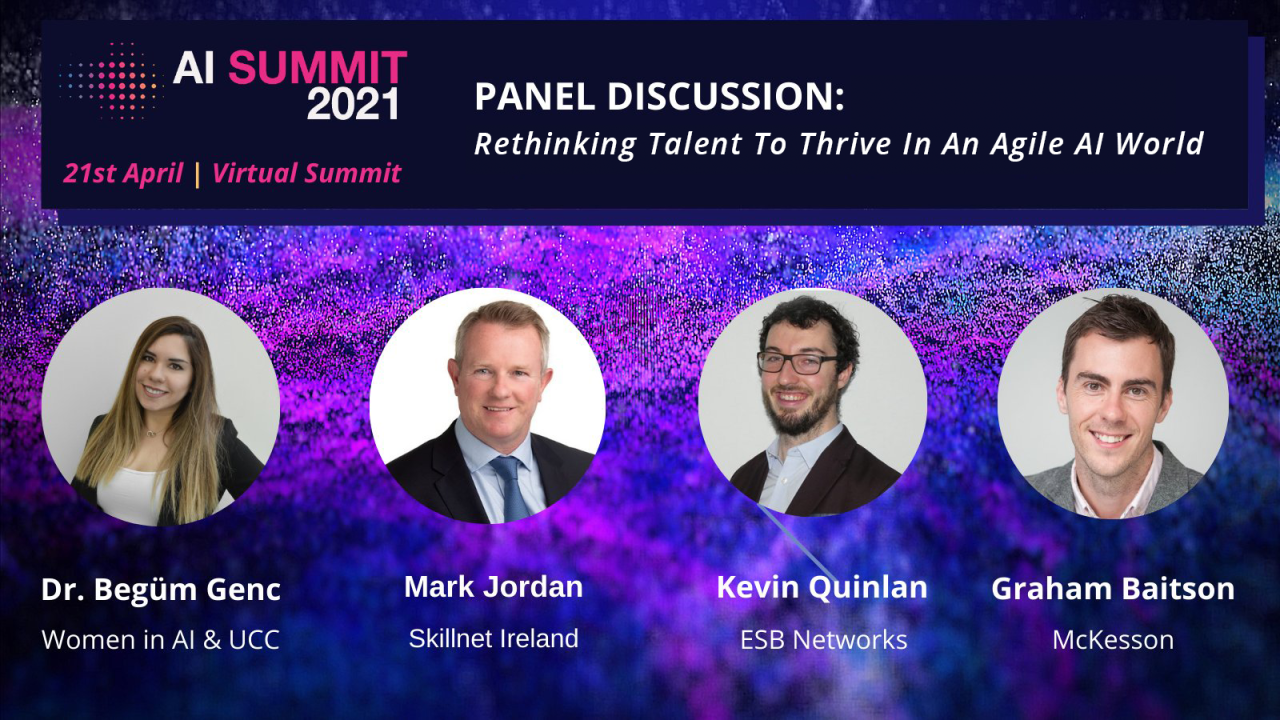
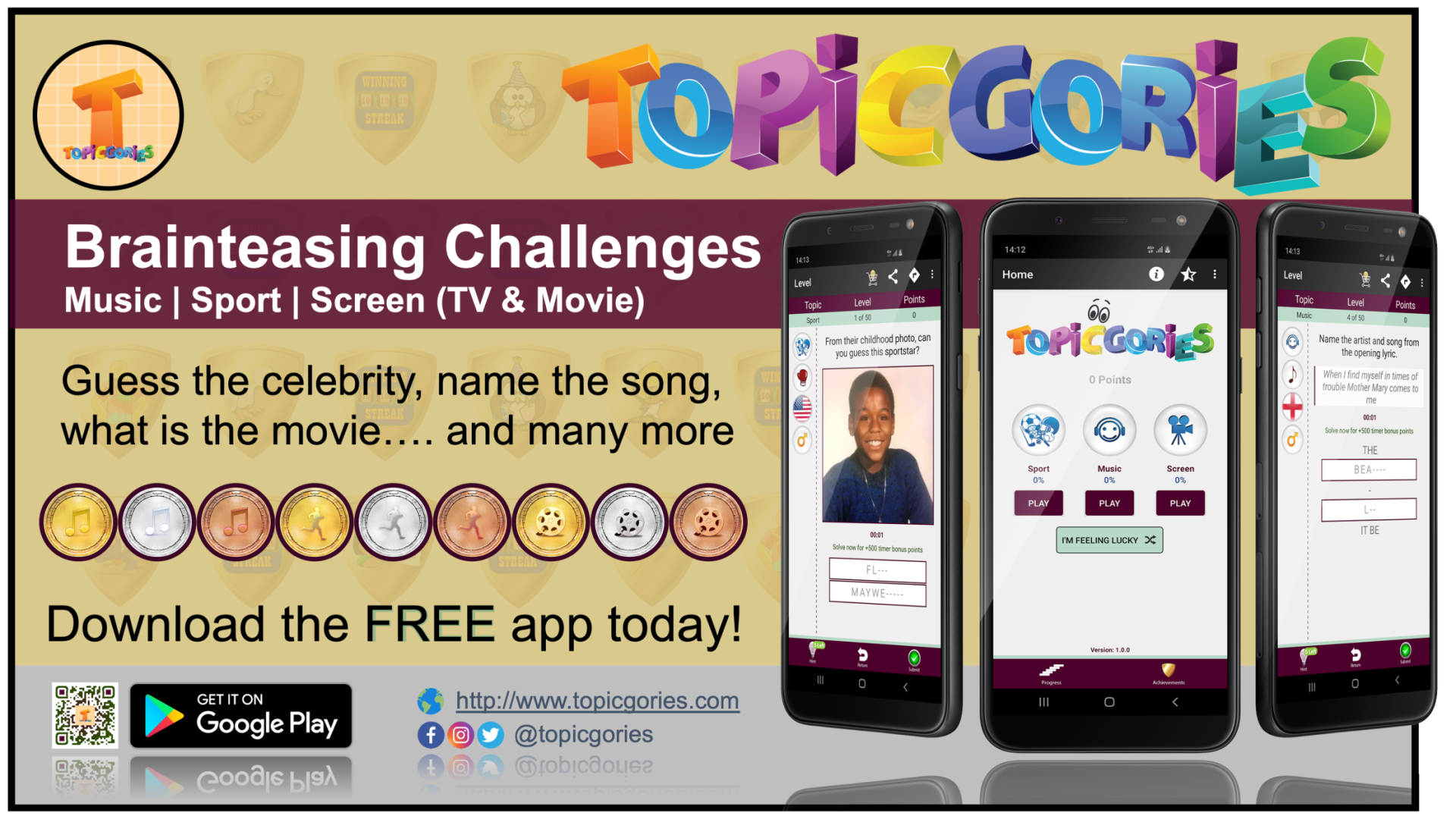